Speaker Bio & Abstract
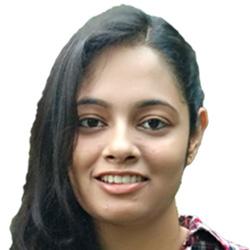
National Remote Sensing Centre
India
BiographyGurneet Kaur is formerly a student of UIET, Panjab University, Chandigarh where she pursued her Bachelor of Engineering (Hons.) degree majoring in Computer Science. She spent her last semester pursuing a research internship at the prestigious Indian Space Research Organisation. During this period, she worked on replacing traditional satellite object detection methods with newer deep learning-based approaches. She is currently working at Adobe as a software developer.AbstractGurneet Kaur, S. S. Raja Shekhar | National Remote Sensing Centre, Indian Space Research Organisation
Over the past few decades, satellite images have been employed in numerous fields, including geography, land survey, earth sciences, military, intelligence, economy, planning and humanitarian domains. These applications require the extraction of high-level knowledge from satellite imagery which is gained through identification of various objects such as mountains, forests, water bodies, buildings, roads, etc. Challenges faced in object detection include non-trivial noisiness, blurring, overlap, non-uniform illumination and textural discontinuity in objects. Traditional detection techniques involve the use of complex spectral features. Not only does this create a dependency on domain expertise, but models developed do not generalize well to higher resolutions.
The present work overcomes these limitations by employing Faster R-CNN, a state-of-art deep-learning based method for multi-class classification as well as object localization, with Stochastic Gradient Descent with Momentum (SGDM) as choice of training algorithm. Models were trained to recognize objects of different scale on a myriad array of satellite as well as aerial images ranging from 25 m to better than 0.5 m resolution sensors. Mean average precision (mAP) values ranging from 0.97 to 0.54 were obtained during testing for these datasets, which were significantly higher than values obtained using traditional machine learning-based techniques.