Speaker Bio & Abstract
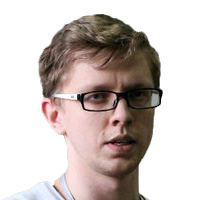
EOS Data Analytics
Ukraine
BiographyGeographer. Had experience in different GIS-related projects: scientific, business, community-based etc. Currently involved in EOS Vision as the Project Coordinator.AbstractLocation data analysis has recently been pushed forward by new technologies. The ability to display and style huge datasets in a browser has shaken the GIS and Data Science communities. Data Analysis became more interactive giving users plenty of options to visualize the data. Therefore, not only GIS specialists could perform the geospatial analysis to discover patterns in their data.
Unfortunately, visualization approaches (heatmap, clustering, choropleth maps (even using an appropriate way to distinguish classes)) are weak in discovering spatial patterns. There are algorithms developed to face such challenges: Nearest Neighbor Analysis, Quadrat Analysis, Local Moran's I, Getis-Ord Gi* etc. They answer the question if features in a dataset distribute randomly, whether these events of processes are geographically determined.
The algorithms mentioned are well known to GIS specialist, and mostly new to not-GIS users. The task we challenged is to develop the combination of algorithms imitating the workflow of GIS specialist for discovering spatial patterns. It combines methods to discover the area of data distribution, data enrichment techniques, choosing the correct algorithm to perform pattern analysis, as well as setting up appropriate hyperparameters to this algorithm. The Spatial Analysis Chain lets users perform advanced spatial analysis and discover hidden patterns in the data.